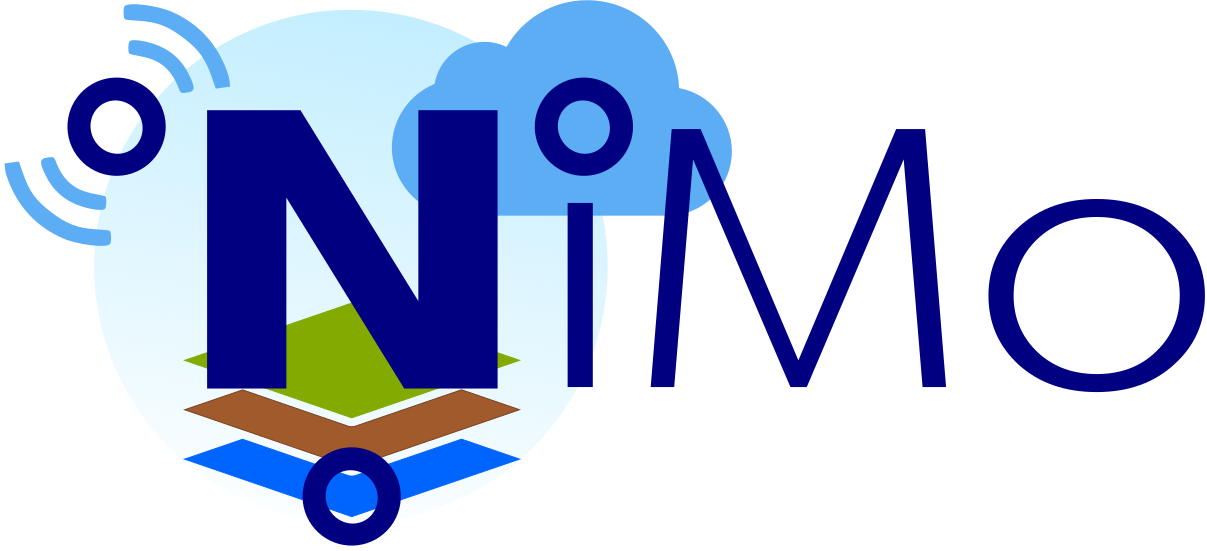
Nitrate Monitoring 4.0 - Intelligent Systems for the Sustainable Reduction of Nitrate in Groundwater (NiMo 4.0)
- Contact:
Dr. Tanja Liesch
M. Sc. Marc Ohmer
M. Sc. Jonas Weis
M. Sc. Andreas Wunsch - Funding:
Federal Ministry for the Environment, Nature Conservation and Nuclear Safety (BMU)
Funding code: 67KI2048C
- Partner:
Disy Informationssysteme GmbH (Disy)
DVGW-Technologiezentrum Wasser (TZW)
Fraunhofer-Institut für Optronik, Systemtechnik und Bildauswertung (IOSB)
- Startdate:
Sep. 2020
- Enddate:
Dec. 2023
Project description
The distribution of nitrate in groundwater is the result of a complex interplay of many influencing factors, including, in addition to input, which is determined by land use, meteorological factors (precipitation, evaporation), chemical-physical properties of the groundwater-covering layers, and transport and reaction processes in the groundwater itself. Nitrate distribution in groundwater therefore represents a highly complex, spatially and temporally highly variable pattern that exhibits pronounced hydro-geochemical differentiation regionally and especially vertically. Although input, transport, and dispersion of nitrate in groundwater largely follow known chemical-physical processes, modeling with analytical or numerical models at a meaningful spatial resolution has been difficult. AI applications, in particular neural networks or deep learning methods belonging to the field of machine learning, as they are often used in other disciplines for pattern recognition, offer significant added value here compared to established methods. As a data-based model, they are able to extract and transfer complex correlations from a large amount of data.
The overall objective of the project is an improved spatial and temporal prediction of nitrate in groundwater and intelligent decision support systems based on this prediction, which contribute to the optimization of groundwater protection programs and thus to an efficient and sustainable nitrate reduction, e.g. through scenario calculations. The considered solutions and methods are developed, demonstrated and validated on the basis of real data from two water management important pilot regions, with sufficiently large hydrogeological variability, in order to be able to make statements on the general validity and transferability of the developed solutions. Furthermore, the spatial prediction in combination with modern methods of geostatistics and operations research also allows recommendations for the optimization of the monitoring network.
Funding
Funding: | German Federal Ministry for the Environment, Nature Conservation and Nuclear Safety (BMU). |
Funding Program: | AI Lighthouses for Environment, Climate, Nature and Resources. |
Funding line: | Application orientation and foundation |