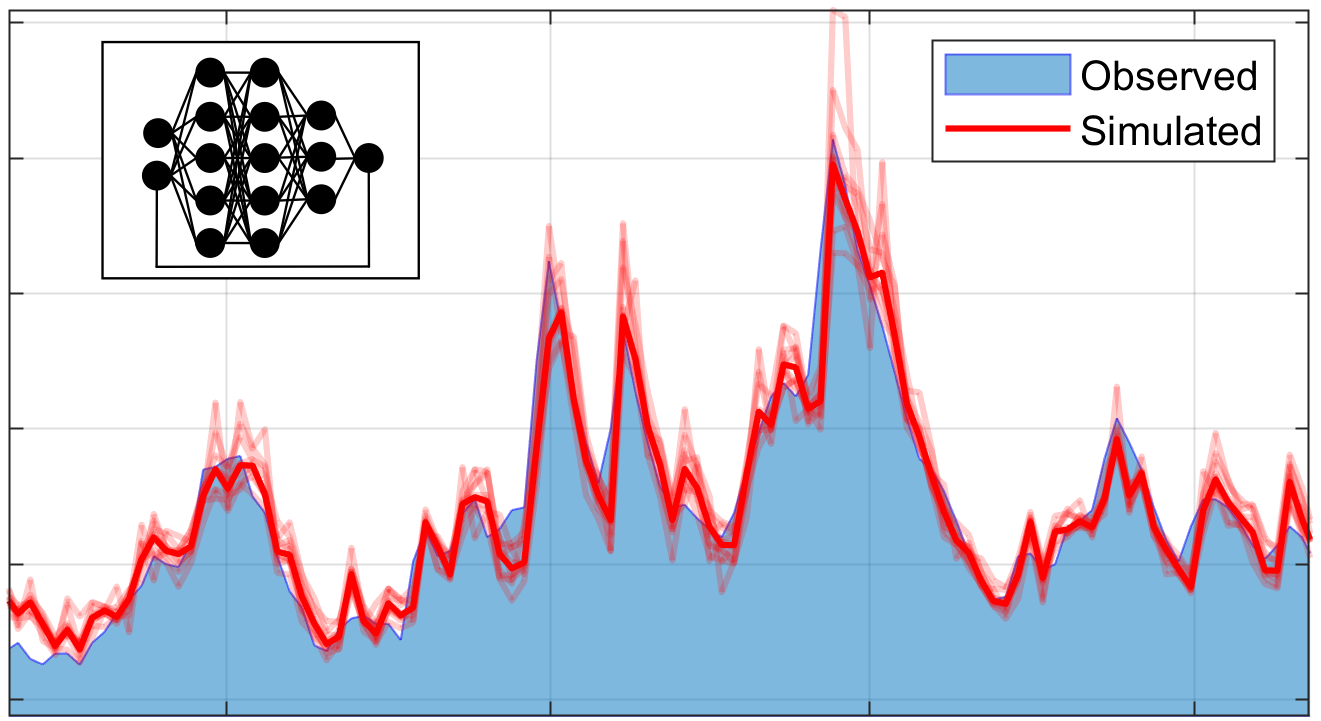
Machine Learning based nationwide groundwater level prediction (MENTOR).
- Contact:
- Funding:
Federal Institute for Geosciences and Natural Resources (BGR)
- Startdate:
Sep. 2020
- Enddate:
Aug. 2027
Project description
Data-driven models, especially artificial neural networks (KNN), have proven their suitability for modeling and predicting groundwater levels many times. In particular, the reduced effort and dependence on extensive field data availability characterize KNN compared to conventional numerical-physical modeling approaches, especially when supra-regional considerations are involved. New developments in the field of KNN, in particular deep learning, promise a significant improvement of already existing prediction approaches.
Reliable prediction of groundwater levels is, for example, the basis for deriving water availability for drinking water supply and irrigation requirements for agriculture, delineating potential land-settlement zones due to extremely low groundwater levels in connection with droughts and/or water withdrawals, delineating areas of potential groundwater highs for transport infrastructure, buildings and agricultural land, and developing appropriate mitigation and adaptation strategies.
The aim is to develop a method that enables nationwide short-, medium- and long-term forecasts of groundwater levels and spring discharges, especially with regard to extreme events (maximum and minimum levels). For this purpose, existing approaches based on artificial neural networks, which are already suitable for weekly, monthly and seasonal forecasts at individual groundwater monitoring wells, will be further developed, and new promising deep-learning approaches will be pursued. The data basis includes groundwater data from the state monitoring networks as well as weather and climate forecasts and climate projections from the German Weather Service (DWD) and other research institutions (e.g. MPI-M).
Example groundwater level forecast